Journey from Excel to AI: How Pairzon Takes the Tedium out of Marketing – Lewis Rothkopf
Welcome to It's Marketing's Fault, the podcast where we discuss how to do marketing the right way.
I'm your host, Eric Rutherford, and I am excited because I have with me Lewis Rothkopf.
He is the Chief Revenue Officer of Parazon, an AI-powered customer data platform that helps retail marketers become data experts and supercharge.
audience targeting, customer acquisition, retention, and ROAS.
Lewis, welcome to the show.
It is great to be here, Eric.
Thank you for having me.
Oh, it is my pleasure.
I'm excited because as I read through, I read through your information, I was reading about Parazon, I'm like, man, this just looks fascinating.
And so the opportunity to just dig in and ask some questions and just listen, I'm pumped.
I know the audience will be as well.
Well, let's just kind of start off with what is Parazon?
Yeah, great question.
So if you think back to the past 20 plus years of digital advertising, ad tech, martech, one really important problem has yet to be solved.
And that is understanding the impact of your online efforts, whether they're display or video or SMS or email, on your in-store offline real world sales.
Very difficult.
Typically, some degrees of In some cases, they'll measure football at a certain GPS location.
The problem with those techniques is that they're not deterministic and precise.
So let's say I'm running a campaign and I want to see how many people come to the store.
You could easily do that with geo tracking.
However, what if the store is in a mall or what if it's the other end of the strip mall?
And what do they do when they got into the store?
Do they buy the products?
How much did they buy?
Do they have a loyalty program member, et cetera?
So it's much more precise and much more dynamic of you into what's happening from my online advertising and when it goes out into the minds of consumers online and offline.
This is all backed and powered by artificial intelligence, which serves to predict for our customers which of their consumers are most likely to convert off.
Wow, so the AI helps predict which consumers are most likely to buy offline.
So is that on a percentage basis?
How granular does that get?
it gets down to the anonymized individual consumer level.
So if I were to segment out my entire customer data file, I would see that at the very top, there are those customers who are champions.
You can't lose them.
You really want them to stick around.
And then it sort of trickles down a bit all the way down to the bottom with folks who maybe haven't been there in a year, maybe only spend a few dollars when they go there.
And you're able to uniquely target or anti-target any of those segments or any of those positions in your customer database.
What that allows you to do is to reach consumers individually, again, anonymized, but to reach consumers individually and to put them into buckets that are based upon their behaviors in the past.
The best part about this, I think, is that it all uses first-party data.
So there's no third-party data that you have to buy.
There is no cookies, third party cookies issue.
This is 100% the marketers first party data.
And so you're not having to do any probabilistic magic.
You are deterministically understanding which of these customers bought something in the store after they saw something online.
It could also do with what, you know, Buff bought something online too, but that's less of a problem.
No, that's brilliant.
Being able to keep it first party data, you're not having to cross data boundaries, so to speak.
It's all in house.
And I like how it's anonymized.
So you've got privacy protections, you've got other things.
So it just makes it safe.
At the same time, it gives you that granularity, which so often we just don't have.
it eliminates some of the guesswork.
It is, and I wish I could show you the platform, but there's a dashboard.
It's the first thing you see when you log in, and a lot of our customers call it their morning dashboard because it is just a very, very straightforward overview of everything that's going on in your business.
How many customers use your loyalty program?
How many customers match between your database and trying to reach them on one of the WaltGarden media buying platforms?
What we're able to do, very, I think, uniquely, is to take all of that first party data through our direct integrations into several of the PLS platforms and then push that data.
Once those audiences are created, we push them to the media buying platforms, to Google, to Meta, to TikTok, so that those audiences can be activated on the retailer's media buying platform of choice.
Wow, okay.
I love that because that is the gap that we so often have.
So what makes Parazon better or different than other like omni-channel marketing platforms?
You're like, it could be HubSpot, could be ActiveCampaign, you know, just any of those.
What makes it that better, different position?
that, yeah, of course, it's that AI driven prediction.
So it's very easy to, and it's funny, people ask who our competitors are, and I think our competitor is an Excel sheet.
Because what we find is that most retailers are trying to get to this information, but the only path they have is to look at extremely granular and detailed data, and then put that into a worksheet, or put it into some visualization tool, and kinda sorta do.
what it takes a machine, you know, bare moments to synthesize.
I like that as a former Excel person who did a lot of data analysis and reporting, and that was the tool available.
I get it.
So really, that's an interesting comparison.
The idea of, okay, you're taking in-store versus a big database.
You're just trying to give...
to take the labor intensive parts out of it.
That's a really great point.
It sort of erases the tedium of trying to do this manually.
What we typically see is when you try to do it manually, you have a big team of data analysts, you have people who are Excel experts, you have people who are CDP experts, and they're having to spend their time to come up with these correlations and these recommendations and generate these audiences.
It's just not practical, particularly if you're a small or mid-sized merchant and you don't have that 10, 15-person analytics team.
you want to get the information you're looking to get in a simple and straight That is so true.
It is incredibly labor intensive.
And then you hope the answers come out right and your Excel file doesn't crash or get corrupted or and sometimes it's just too much data for Excel to handle.
That's right.
Wow.
So what can AI solve for marketers?
What kind of marketing challenges does it do well?
And where does AI not do as well within this sphere?
Yeah, so I'll answer that in sort of a weird way, but I will get to it.
If you think about marketing and sales in a organization, these two departments tend to be really siloed and isolated from one another.
And that's why you have marketers optimizing their media to things that quite frankly don't matter, like click-through rate and cost per completed view and so forth.
These are things that are having really no impact on whether a consumer is actually going to buy the product or service that's being analyzed, excuse me, that's being advertised.
And so when you get this bridge together between the departments of the business, typically sales, marketing, and technology, you're able to say, look, let's work off of a common sense of set of numbers.
Let's use the same data between sales, marketing, and technology that we really can get a better view on what's happening.
You can use natural intelligence.
and a team of lots of people to try to pick out these insights, but you won't do it nearly as quickly.
And any human, even the smartest analyst, will miss correlations and will miss predictions that machine learning algorithms are able to generate.
So that's fascinating.
And as a marketer who's worked in large companies, it is, it's siloed.
You've got marketing, you've got sales, and occasionally they meet, but usually they don't.
And that is a problem, right?
It's like, as marketers, we do the best guesstimate in many cases of how to do attribution and what's going on.
And then you made a really interesting comment on click-through rate.
Right.
Would you elaborate a little bit on that?
Because I think as marketers that in some ways, we think of that as maybe not the Holy Grail, but very much one of those, we can't argue with click through rate, or we have to have click through rate, or that's like the pinnacle.
Would you elaborate on what makes it not that helpful?
Yeah, so you could argue about click through rate.
We still do it today, but 25, 26 years ago when this industry was really beginning, we made a huge mistake and we planted this seed in advertisers' minds that click through rate was the metric, right, the KPI.
And so what a pollsters do, they optimize to clicks, right?
If the KPI in this campaign is click through rate, well then let me optimize this campaign to the sections of my site.
that have the consumers that are most likely to click.
The problem is studies have shown, going all the way back to the beginning of the industry, that there is zero correlation between people who click on ads and people who actually buy stuff.
In fact, what a couple of these have found is that people who click on ads are explicitly not, in general, the people who buy stuff, statistically.
So if you're optimizing to a metric that you feel comfortable with, because everyone knows what click-through rate is, then you're missing everything.
You're missing the sales part of it.
And that gets back to the siloed nature of marketing, sales, and technology.
So if you've got a marketing team saying, hey, my OKR for this quarter is to establish a 1% click-through rate on all of our advertising, well, now you're driving your business towards a 1% click-through rate.
You're not driving your business towards sales.
And at that point, what are you doing and why are you doing it?
Because that's a little crazy.
Another one that folks use is video completion rate.
So how many people took the time to complete this video before clicking away and moving on to something else?
Well, the problem is if you're basing your success of the campaign on video completion rate, then you're incentivizing the suppliers to run your video in a way where it's going to be completed almost no matter what.
So maybe that video is playing below the fold on a web page, or maybe that video is running on a CTV and the television's not actually turned on.
So you really have to let it, it happens, right?
You really have to look at what is the real world impact of advertising on my business?
Because when you look at these older or proxy metrics or less generously called vanity metrics, you're just not driving the business forward.
It feels good, but it's not accomplishing anything.
That's fascinating, and it really is.
And is it we've just gotten stuck in our ways, or is it because we've just, it's an easy thing to measure in terms of how our systems are set up, whereas the moment you get away from it, attribution gets a little bit murky and gray and a little bit harder to come by.
I'm just curious.
Yeah, it's very interesting.
When you look at sort of the deterministic side and understanding what happened in this campaign, it is very easy for you to measure impression and clicks.
It's the same thing we did in 1999 as we do today, right?
Impressions and clicks.
So I think it sticks around because it's really ingrained in people's minds, right?
Agencies are evaluated based upon metrics like...
foot for rate and cost per completed view.
And at the risk of sounding like a broken record, this is why it's so important to have a connection between sales and marketing.
Because you've got the sales organization worrying about how many products are sold, which is kind of an important thing, to get the marketing organization trying to optimize to marketing metrics.
And it's just as imprecise as heck when you're not tying your advertising to sales.
It sounds, you know, elementary and obvious, but it's very uncommon.
Wow.
No, and I appreciate you going into that because, you know, it's, we are bombarded and measured by click through, you know, view duration, all of those things.
It sounds like we've, you know, we're trying to climb a ladder that's up against the wrong wall.
It's like, what are you doing?
And I think if you were to pull one of these folks into the coffee break room and say, what are you doing?
This is crazy.
They would say, well, it's in my goals to have a high click-through rate.
And then they would say, it's in my boss's goals.
It's in my agency's goals.
And then all the way to the client side, the marketer has a goal of click-through rate improvement.
And maybe you'll get some signal from it, but it's just too divorced from.
the real world to make any, I would argue, to make any media planning decisions based upon.
Now, wow.
I think that's, I appreciate you going into that.
I appreciate the insight, because that makes total sense.
And then, so let me ask this, what makes it so important for marketers now that we've got the AI technology, now that it's going, you know, as it's iterating and it's more available, you know, to...
I'll say non-techie people for lack of a better description.
There's just a lot of opportunities there.
What makes that AI so important in order to predict best customers?
Like, just, I'd love to hear your thoughts on that.
Yeah, so what we typically do is ask our customers for one to two years of transaction data.
So we'll use that data to train the AI on what are these customers doing?
What is the correlation between products they bought and adjacent products on the shelf or online?
How much are they spending?
What are they getting paid with?
Is it credit card?
Is it cash?
Right.
And so all of these data that are taken into the AI and sort of turned through, talk, you show me what's happening with this customer's client base, show me what people are doing, how often are they coming into stores?
And we'll use marketing models like RFM, recency, frequency, and monetary value to sort of stack rank these consumers and then predict which of them are most likely to become customers or which of them are most likely to come in store and retain themselves as a customer.
It's also very important to do the opposite, right?
So I think One thing that everybody who has ever used the internet would say is, I was shopping for a pair of shoes and I went in the store and I bought my shoes, but I am seeing that shoe ad everywhere I go on the internet.
It's crazy.
It annoys consumers, but perhaps more importantly, or as importantly, you're wasting money.
Cause if you're running this ad to someone who has already bought the shoe, what are you doing?
It's literally money that you are wasting.
And so targeting based upon those who are likely to convert and then D targeting those that are not likely to convert perhaps because they just bought something is critically important in managing your media budget, right?
It's the old, um, want to make your quote of half my advertising is work.
There's no reason for that to be a thing any longer, right?
There's, there's no reason to not know precisely which of your advertising is working so that you can optimize it and manage towards it.
I think that's so important.
I'll keep the company nameless right now, but I have gotten emails from companies on products that I have recently bought, asking if I want to buy, essentially buy the same thing again or something similar, but it was a one-time purchase, right?
What I was buying is not like something I'm going to buy once a year.
Great.
it might be like once every 15 years or because I had an emergency or, and I'm always baffled by those.
I'm like, that seems like a really bad, a bad connection point.
And it feels like it's very much the same way with when you're thinking about ad spend, it's like, don't, it's one thing if it's a reusable, disposable type of item, but like if it's a car, if it's like, kitchen cabinets, if it's like, you know, any of those type things where it's a very infrequent buy, it's like, man, you're, it's a waste.
It's, it's, it is literally a waste.
You know, unless to your point, you're talking about something that has an insanely fast refresh rate, right?
Like a thing of razors or a thing of milk or orange juice or what have you.
But if you're buying a mattress, you're probably not going to buy a mattress again for other 10 to 15 years.
So for the love of God, let's stop advertising a mattress to this guy who just bought a mattress.
The problem is if you don't close that loop, right?
If you don't connect your online advertising.
to your offline in-store sales, you'll never know that.
You'll never know that guy bought a mattress so you should stop advertising to him.
And you're also getting sort of bad signal in your analysis of, well, this mattress campaign isn't working because we're showing it to people who are mattress intenders, and I'm only seeing a 0.01% click-through rate wise up because you're showing it to the wrong people.
Either you're showing it to the people who have no means and wherewithal to buy a new mattress, or you're showing it to people who have.
either from you or your competitor.
And as you said, that is just literally money that you're pouring down the drain.
Wow.
And that it's, it's when, as you describe it, it really is just a closing the loop on making that connection to be able to omit these particular audience customer people, ultimately saving your money, making your system work faster and making your numbers more accurate.
Yes, now that's not to discount the impact of branding campaigns on digital advertising, right?
And so, yes, you want to remain top of mind, but I am a believer in the axiom that all advertising is performance advertising.
Even if you call it a brand campaign, the agency, the marketer is still backing it out to direct response ventures.
So branding is important, but again, when you're focusing on your mattress sales store, you should probably put this person.
out of your active column for what, five years at least, six years, if you are a co-location company, maybe it should be one year, right?
And if you are a grocer, a lot of our customers are grocers, it could be as minimal as a day or week, whatever the refresh cycle is on groceries.
So it really comes down to product type, on who your customers are, what their purchase patterns are like, and then just go, it's not like an industry standard, it's very much unique to your business case.
And that gets into your previous question, right?
About like what happens when you take all these data, you mush them together into an AI and sort of answers get spit out.
How are those answers spit out?
It's by analyzing those years of transaction details, along with ideally a live or daily feed of your ongoing transactions specifically so that you're able to move people into different buckets of marketing and you can create a segment out of any bucket.
You can create a segment and push it to the media buy that consists of really strong customers or really not strong customers who need activation.
You can also create segments based upon any dimension that you're collecting at the cash register.
So color of the item, price of the item, is the consumer a loyalty program member?
Do they live within 25 miles of the store?
So all of these things are taken in concert in a way that humans really can't do.
And that's the...
and the benefit of AI, I don't think.
I'd agree.
And that was something in the past when I was trying to do some attribution and other things like that, I always felt like I needed more levels of understanding.
Like, if you go back to that Excel example, Excel is two dimensional.
I felt like I needed like five dimensions almost, just to be, because there's no way to...
even with like SQL and other things, there's no good way to line some of this up to get those attribution answers.
And so it sounds like with some of the AI programming, with some of the AI options out there, what you were able to do then is really take what you couldn't physically line up and now connect the dots.
That's exactly right.
And you make a good point for years, trying to get data out of your database, such as this, such as your shopper database requires that you learn SQL, right?
And it puts together these queries and it's usually like one person at the company who knows SQL and you've bothered him or her all the time within our platform, it's all simple English language Boolean, right?
So I want to reach people who are loyalty program members, right?
Who do live within 25 miles of the store and who do have children.
And I explicitly don't want to target people who have bought a product from me in the last month.
Making these up, but you can create those segments and then segments of segments, see the size of that audience, if it's significant enough, push that audience directly out to your media buying platform to activate.
Wow.
So it's almost like, I don't know if it is just almost like point and click and drag and drop.
Well, okay.
That's brilliant.
That is light years ahead of running SQL code and everything else because it puts it in the hands of people who don't have to be so technically focused.
That's huge.
How?
How can you, I guess, let me try this question.
So this idea of look-alike audiences, I had seen that.
What are they and how are they helpful?
Would you just go into that some?
can, I wish I had a whiteboard so I'll see if I can do this in the air.
So you've got, it's called a seed.
You've got your deterministic match of this consumer likes to buy.
Soy milk, right?
And so now you know, but do you, do you do a query of your database?
When you see that only a thousand consumers really making these off really like soy milk.
And so that's not really big enough to have a ad campaign against.
You might've built this.
The old joke is pick up your phone and call those thousand people and try to sell to them.
In order to make critical mass where it makes sense to run a media campaign, we look at lookalikes as a model of people who express similar attributes to those that were in the seed population.
Now, how similar and how precise is really where you have to make a decision between accuracy and scale?
So you know those first thousand people.
You literally know.
that they bought soy milk in the last month.
You don't know all the other people on the internet, but you can say, I wanna find people who exhibit many of these same behaviors and many of these same attributes.
Now I mentioned, you're sort of facing the tension between accuracy and scale.
So you wanna reach 100,000 people who may like soy milk.
Yeah, like you can use that seed population to build a lookalike audience of people who look like people.
who buy soy milk, but once you get to 100,000 people, a million people, the number of people that you're identifying incorrectly is going to increase.
And so imagine a slider all the way at the end of accuracy and then all the way at the other end scale, and you just have to pick the point that you're most comfortable with as a marketer between accuracy and scale, such as saying, maybe this is 90% accurate, but it's worth it.
It's worth losing that 10% if I'm hitting 90% of my target consumers anyhow.
That's fascinating and it sounds like then it could depend on the product and maybe the revenue from that product as to where you want to be on that sliding scale.
So if you're selling higher end items, it's okay to have a slightly bigger audience simply because the money you will make off those sales will recoup your ad costs versus if you're selling soy milk, you want, you need a narrower population.
Is that something where you, is that like trial and error where you're like, okay, I'm going to look at this.
I'm going to take my best guess scenario.
Let's say that I want 90% similarity.
And then you try that and then you say, okay, that either I want more or the same or less.
Is that how that works?
That's exactly right.
So you take a look at your seed audience.
Let's say you're running a campaign and you want it to be a 100% deterministic match campaign.
No probabilistic matching, no lookalikes.
I just want to reach these people.
And because I want to reach these people, I'm going to run a campaign to them.
And because the product exactly to your point, maybe it doesn't cost that much.
Maybe it has a very high refresh.
Let's take a chance and reach people.
Now, if you see a campaign is not delivering at all.
If you just cannot reach the users you're trying to, well, then your campaign can under deliver unless you open up the targeting a little bit to include local legs.
Now that gets you into a situation where you may not have 100% accuracy, but just as you said, you know, maybe 90% is, is good enough.
Now this won't work in niche categories.
So let's say you are a business jet company advertising your jets.
And let's say there's.
a finite population of 10,000 people in the U.S.
You notice, you notice research, and it's from your experience, 10,000 people in the U.S.
who have the means to buy a business jet.
In that case, you probably would not want to do lookalikes because I don't look like somebody who could buy a business jet.
My behaviors are not similar.
I look like somebody who buys soy milk, but because I'm not in the market, I'm not capable of being in the market for a business jet, that slider should...
probably be all the way down to precision, right?
Deterministic seed population.
Now, if you want to experiment and kind of move that up to the 10% lookalikes, 15%, you can probably find that sweet spot between scale and accuracy, where nobody expects you to reach 100% of your audience, 100% of the time, and there's going to be some waste.
But the more you sort of fine tune it, and the more you recognize, just as you said, your product that you're selling, your business model.
your value proposition depending upon what that is you'll more or less want to use lookalikes to have a big enough scale.
No, I love that the business jet example too, because that's like there's when there are many businesses who there are just a fixed amount of potential customers and you can't wish more into existence even if you had like a magic lamp.
It just doesn't work.
There's only so many.
And so going trying to go beyond that usually isn't helpful.
Yeah, yeah, you can do it.
And the media properties will be happy to run it for you.
But you're just not going to get your money's worth on those whom you know don't have the wherewithal to buy a business check.
So, what about things like, I'll call it for lack of a better term, bundling of products.
You think of your basket, what goes in the basket, your average basket, things of that nature.
Does that also play into how this works in terms of trying to look at purchasing decisions and being able to do that targeting of marketing and ads?
Or is that...
or does that totally skew the models?
Nope, you're 100% correct.
So probably one of my top favorite features in our platform is a visual explanation of the relationship between products that you sell.
So I'm gonna see t-shirt, and then I'm probably gonna see a line from that t-shirt to underwear.
Okay, that's pretty intuitive, and maybe you have your t-shirts next to your underwear.
But what if I also see a correlation between t-shirts and blue sneakers?
Well, I didn't know about that.
I mean, that's the sort of thing that a machine can pick out.
It probably makes sense in that case for me to put t-shirts next to blue sneakers in my store, because why wouldn't you?
And then online put t-shirts, when someone puts a t-shirt in their cart, I'd like to recommend that you also consider these blue sneakers.
Again, it's all about taking the data that you as a marketer have and just squeezing every little bit of use out of it.
And those uses are not always immediately apparent.
That's fascinating.
I mean, that is so fascinating, and it just sort of blows my mind because those were some magic numbers, right?
That 10 years ago, you couldn't find.
Like they just weren't there.
It was not possible to be able to get that attribution.
And so I imagine then, a lot of the businesses who work with you, for lack of a better term, it's almost like they, they find this surprise correlation between items.
Any examples of that?
I'm sorry, I'm geeking out.
I'm having a moment where I'm like, that's brilliant.
Oh my word, I'd love to hear more.
Many of the examples are...
evidence right so things like t-shirt and underwear that's not really surprising i don't have a specific example off the top of my head but let's say it's back to be the example of the t-shirt and blue sneakers you probably would not know that even if you are a expert merchandiser even if you know run your store uh you know tight ship you're still probably not going to know all of those correlations but why not put those correlations to work for you even if you don't understand at an why t-shirts are associated with balloon sneakers.
They are, for some reason.
So take advantage of it.
true.
You don't have to know why.
You just need to know that it happens.
It happens.
And then I'm assuming you can throw seasonality into that mix as far as, you know, even on a, I don't know, like week, you know, prior year to current year from a day by day, week to week basis as well, that you can add another layer of complexity on that depending on your product mix.
That's awesome.
Wow.
Okay.
My head's spinning.
This is just so cool.
Let me ask one other question because as we're dealing with tech companies, as you're dealing with AI, this is much more than a...
I mean, this is global in terms of reach.
What challenges or advantages...
It's just different sides of the same coin.
is there with bringing foreign tech startups to US customers?
Because I know there are like a couple of like key words that sort of send panic through some people, not all people, but AI is one of them.
Some people are fine.
Some people go running and hiding in their closets.
If you're in the US, there are some people who are fine with globalization, others who get a little nervous.
So what are some of the advantages or challenges to bringing those in?
What's that look like?
I mean, I'll use ourselves as an example.
The company is based in Israel.
We have a lot of customers there.
We've been around for about two plus years.
And we're bringing the business into the US exactly as you asked.
And so my charge is to bring the same quality of information that we brought to our customers in Israel and Europe into those in North America.
The opportunity is you get to say, let me take a step back.
It's very difficult as a new company, as you know, to get people to take part.
you're selling.
You'll say, let's do a pilot well, who else are your customers?
It's very, very difficult to launch a business in the US, especially from a cold start.
The benefit that we have, for instance, is that the business has existed in Israel for the last two plus years in Europe.
And so it's been put through its paces.
Many US companies, their local Israeli equivalents or the local Israeli divisions are our customers.
So there's exactly, there's a lot of that connectivity between what did we do, what did we learn in the lab in Israel?
And as we commercialized in Israel, what did we learn there?
And what are the bugs that we saw?
What are the problems with the models that we saw?
And now that we've done all of that learning over the last two, three years, it not only benefits our current customers, but it gives us a lot of strength when we come in the US and say, we're new to you, but we're not really new.
And here's sort of a list of the key studies.
Now, To get back to your core question, which is, is AI scary and is it scary when it comes from a different country other than where the marketer lives?
And I mean, is the internet scary?
The internet's a little bit scary if you don't use it the right way.
That's why there's laws and that's why there's regulation.
AI, of course, is badly regulated, not regulated today.
So I think you're going to see a world in which just as there are laws around consumer privacy online and child protection.
I think it's fairly obvious that we will see legislation around AI and the permitted uses and not.
And just like anything else, you're going to have companies that are good corporate citizens and don't use AI for nefarious purposes, and then you're going to have bad actors who do.
And it's just a matter of differentiating between good and wasteful or bad AI.
I'm not one of those people who believes that the robots are going to come and rise up and take over society, but AI is only as good as the data you're putting into it.
So you still need to make sure that the input and the training is accurate and useful, or you're just gonna get garbage out.
And that's a good point.
It's just a tool, right?
AI is just a tool.
It's like a hammer.
It's like whatever.
It's just neutral.
And so it's, yeah, it is.
But what you said about the training of the data, garbage in, garbage out, that's the other thing is just being very cognizant of what's going in and making sure that it's good.
Because anybody can create...
forever.
You can create a spreadsheet, you can create a database, you can do whatever.
But man, messy, dirty data doesn't...
I mean, it's just a nightmare.
So that makes total sense.
But I like too how you described...
You guys, Parazon's been in business for a couple of years in Israel.
You've got a footprint in Europe.
So you're not trying to just get new...
customers.
You've got that social proof.
You've got the references to show, hey, these people who are very similar to what your company is, they're having great success.
And I'm sure too, as you deal with global companies, some of them are probably already working with those same companies in Israel, in Europe, and everywhere else.
And so it's a really easy...
a really easy sell, so to speak, of getting them to adopt.
Something worth mentioning along those lines is we are predominantly a cloud-based company.
Your data is segregated from other customers' data, but it takes place in the cloud.
For some of our customers who might be financial institutions or might have very, very stringent requirements about use of data, we can also install the platform on site.
So we have an on-premise model, same capability, same pricing, same everything.
It just lives in your server versus somewhere on the web.
Wow, that's significant because especially with, depending on the data that you're trying to understand, eliminating the transmission to the cloud definitely can add that privacy security functionality.
It sounds like just at the same price, that's like, wow, okay.
That's huge because sometimes on-prem can be.
more expensive, more of a headache.
It's just more work usually.
So that's huge.
Let me ask, just as we're wrapping up here a little bit, one takeaway you would like to leave listeners with who are, as maybe they've been listening through and they're like, wow, this sounds awesome.
Any takeaways you'd like to leave with them?
Yeah, so for goodness sake, I hope you work with us.
Either if you work with us, if you don't work with us, I implore you, Mr.
Miss Marketer, analyze your campaigns and optimize them to metrics that actually matter.
Sales matters, clicks do not matter.
And you're gonna get to a point in this industry where there's enough of a groundswell away from these old vanity metrics that you're gonna start to look like you're not as up to date with the current state of the art.
as you were if you were drawing that straight line correlation between marketing and sales.
love that.
So just be on top of your metrics.
Don't fall prey to old, inaccurate thinking.
Just do the best attribution.
Lewis, this has been awesome.
For people who want to know more about you, want to know more about Parazon, where do you want them to go?
So, parzon, that's P-A-I-R-Z-O-N dot com is the website.
There's a link there for more information.
We'd love to talk to you.
And if anybody wants to talk to me personally, it's at Lewis, L-E-W-I-S-N-Y-C.
Excellent.
So for everybody listening, we're gonna drop all that in the show notes, please check them out.
Man, this is like all I'm thinking is through this conversation is where were you ten years ago?
Like like that's all I could think of was like, oh wow, this would have been crazy good when I was when I was doing more of the data side.
So Lewis, this has been this has been incredibly informative for me.
I've loved this conversation.
Thanks for joining me today.
My pleasure.
Thank you for having me.
All right, let me find the...
Creators and Guests
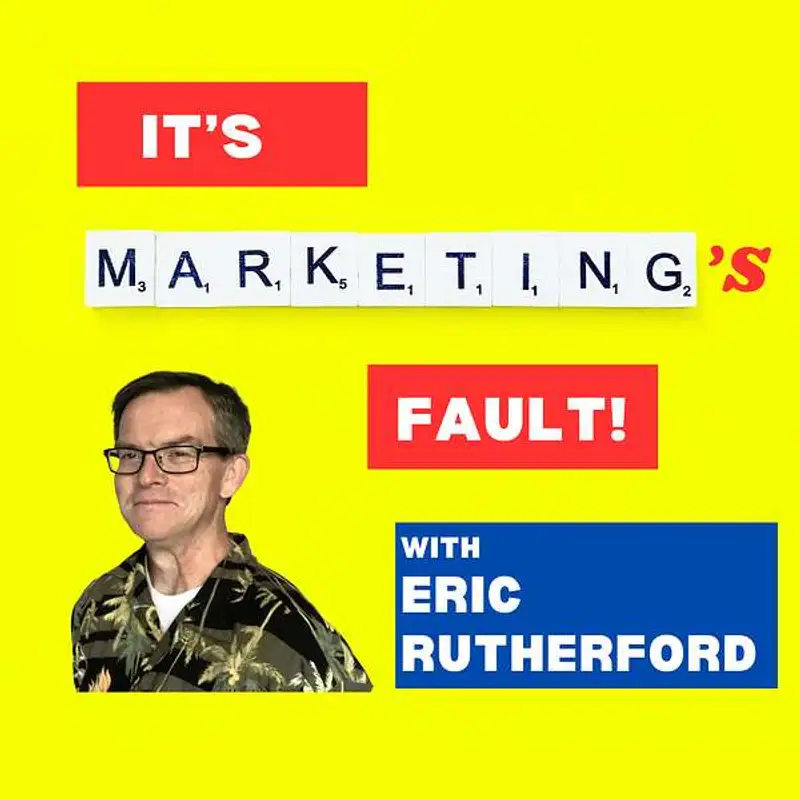